A simple Facebook post can now lead to the prediction of dropout intentions of individuals from addiction treatments, thanks to artificial intelligence (AI)! A recent report published in Neuropsychopharmacology reveals that It is possible to determine if someone will stay in treatment for a substance use disorder by looking at their social media activities. The study also showed that AI does this job more aptly than standard psychological tests.
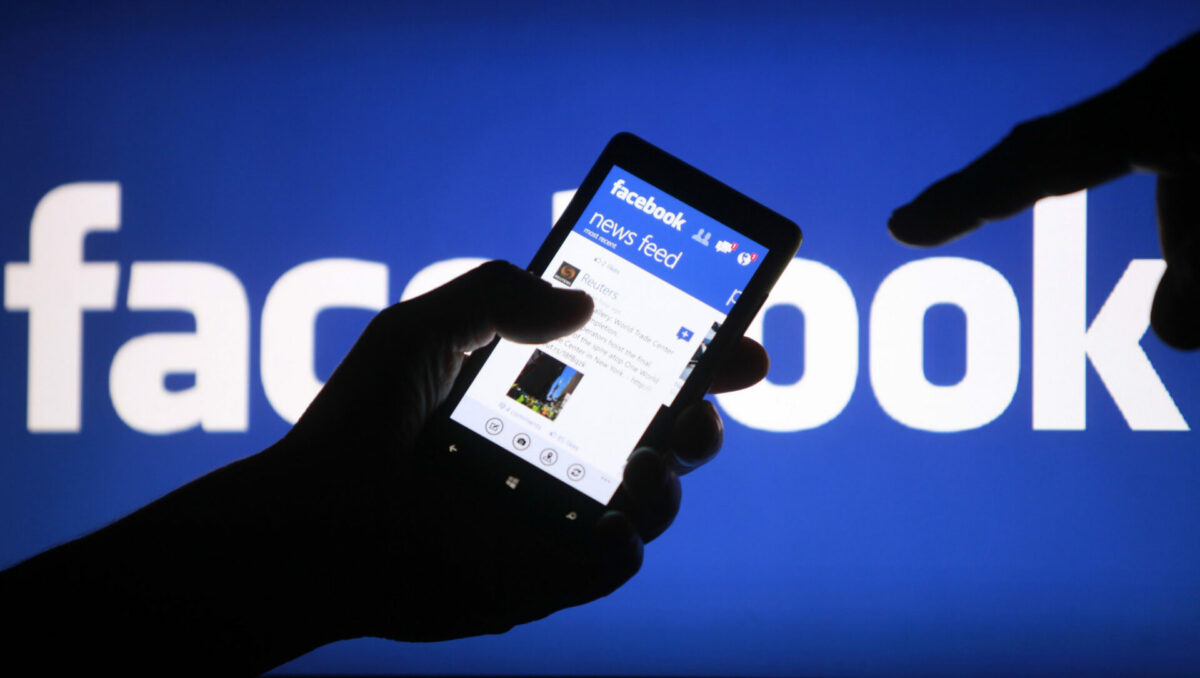
Substance Use Disorder and Addiction
A person with substance use disorder (SUD) suffers from brain and behavior issues, making it difficult for them to manage whether or not they use legal or illegal drugs, alcohol, or other substances. Addiction is the most severe type of SUD, with symptoms ranging from mild to severe. Excessive and compulsive drug or alcohol use, which significantly impairs several facets of the individual’s life, is the hallmark of SUD.
To effectively treat a patient, a variety of therapies, including counseling, support groups, and medication, are typically used in conjunction. However, it’s typical for patients to resume substance usage post-treatment and discontinue the treatment.
Dropout seriously adds to the problem
After examining a sizable quantity of data from 151 distinct studies on SUD treatment facilities conducted between 1965 and 2016, researchers identified that nearly one-third of all participants in drug addiction treatment programs drop out and do not finish their treatment. Dropout rates and recurrence rates are tightly correlated, making treatment abandonment a serious problem.
Identifying the potential Dropouts
Researchers are looking into techniques to recognize those who are more likely to effectively finish therapy and avoid recurrence in order to increase the effectiveness of treatment. One research strategy examines how people with SUD utilize social media, as this might offer insightful information.
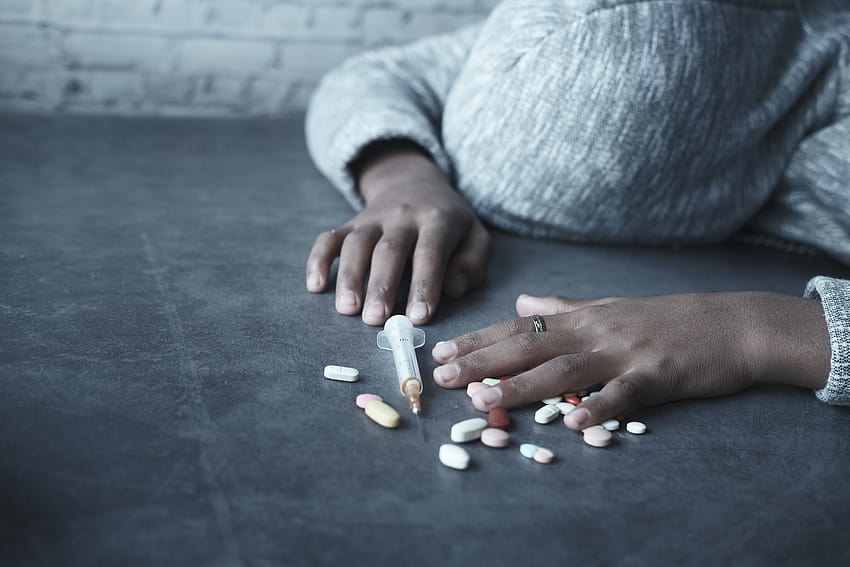
However, it can be challenging to locate such individuals. The self-reporting method, currently being used for the purpose, is not precise enough to accurately identify and evaluate the factors that influence how people will behave and respond to therapy. In this regard, the ecological information gap refers to a person’s failure to comprehend the difficulties of daily life that are important to the emergence of a substance use disorder.
Analyzing the social media activity of those with SUD rather than self-report evaluations may be a feasible strategy to address the ecological information gap. However, compared to self-evaluation, social media activity is way more challenging to analyze.
Digital Phenotype is the Breakthrough
Brenda Curtis and her colleagues wanted to determine if AI created something called a digital phenotype and if it was possible to anticipate how well treatment for SUD would work by describing a person’s digital behavior quantitatively. They examined Facebook postings from a cohort of 504 individuals receiving outpatient treatment for substance use disorders in Philadelphia using an AI tool that can analyze textual data and natural language.
Among these 503 subjects, 269 posts contained enough language information (at least 200 words) for the AI program to produce a reliable prediction of their behavior. Before the start of treatment, projections based on digital phenotypes were created using more than two years’ worth of Facebook posts. The AI tool examined links shared by participants together with free-form text, which is typically shorter than status posts and status updates, which are longer texts individuals post on their timelines.
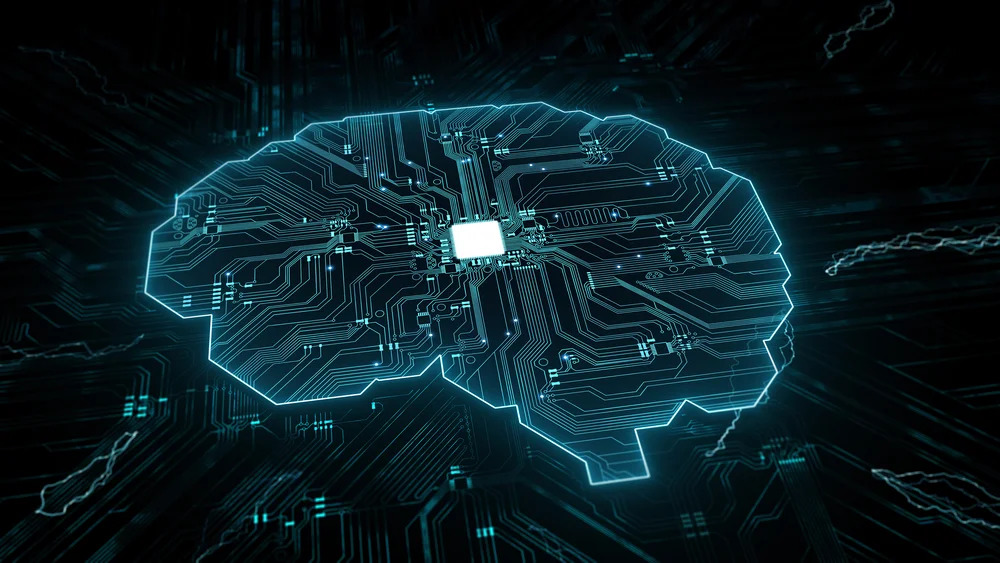
Out of these patients, 269 people’s postings with sufficient language data (at least 200 words) allowed the AI program to produce stable forecasts for their behavior. Prior to treatment, more than two years’ worth of Facebook posts were used to create the predictions (digital phenotypes). The AI technology examined both link posts—links shared with accompanying text that are often shorter than status posts—and status posts—longer text posts on users’ timelines.
AI is the key
The findings of the study revealed that predictions of treatment outcomes based on digital phenotypes were more accurate than predictions based on the conventional assessment of the severity of addiction symptoms. This distinction was especially apparent in adverse outcomes including relapses and treatment discontinuations.