Machine learning has empowered versatile domains of science, technology, industry, and e-commerce with intelligent concepts. In medical and diagnosis, it has garnered several applications that have proven to be more efficacious than traditional ones. A new study published in Nature Medicine demonstrates the same.
The study led over by the researchers from the University of Pittsburgh uses electrocardiogram (ECG) readings as input to their machine learning model. The model is ultimately able to perform diagnosis of heart attacks in a rapid and reliable manner than most current approaches. At times when results from ECG are not discernible, the additional tests like blood tests take a full day to provide with complete preliminary check-up. Also, the expense and waiting time does not always prove to be friendly in severe cases. The lead researcher of the study, Dr. Salah Al-Zaiti noted the brilliance of the model in risk assessment to help patients receive care without trouble and delay.
Training the Machine Model
Medical Practitioners can easily identify a distinct electrocardiogram (ECG) pattern, known as STEMI (ST-Elevation Myocardial Infarction), which indicates the most severe type of heart attack characterized by complete blockage of a coronary artery, thus supply of oxygen-rich blood to the heart is completely blocked out with respect to that artery.
However, the challenge lies in the fact that approximately two-thirds of heart attacks caused by severe blockage do not present a recognizable ECG pattern. To overcome this issue, a promising model has been developed to characterize distinct ECG clues that are challenging for clinicians to detect, thus improving the classification of patients experiencing chest pain.
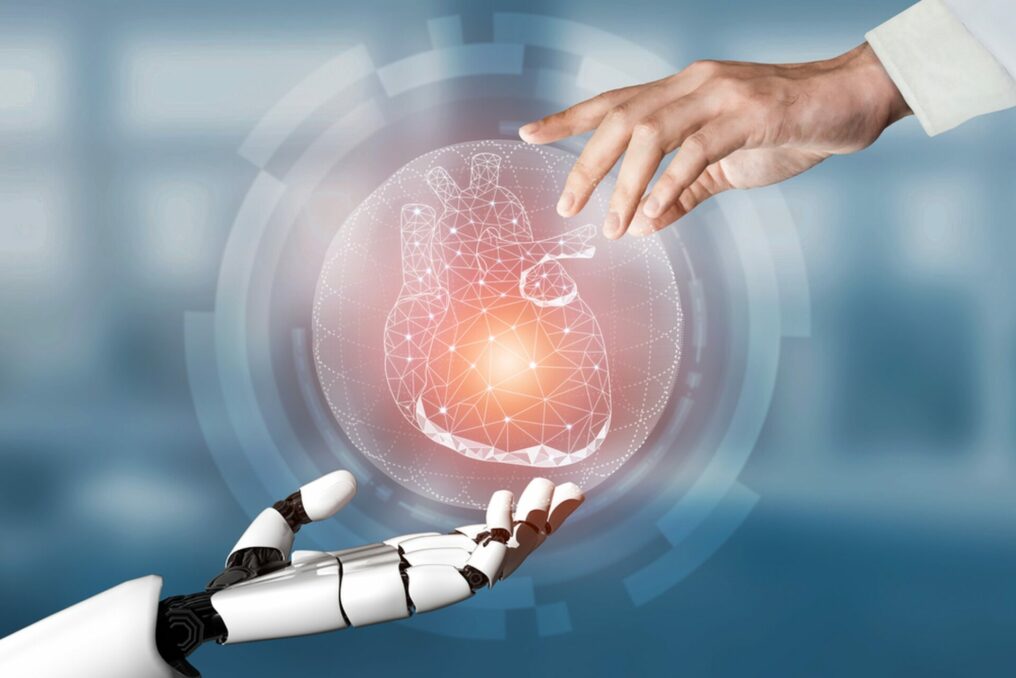
The model was trained using ECG data from 4,026 patients with chest pain across three hospitals in Pittsburgh. External validation of the model was then performed using data from 3,287 patients from a different hospital system.
The researchers compared the performance of their model against three established methods used to assess chronic cardiac events: expert clinician interpretation of ECG, commercial ECG algorithms, and the HEART score, which takes into account various factors such as pain symptoms, ECG interpretation, age, risk factors (e.g., smoking, diabetes, high cholesterol), and cardiac markers from blood tests that measure levels of troponin in the blood.
The model was reported to hurdle out all three benchmarks, accurately reclassifying 1 in 3 patients with chest pain into low, intermediate, or high-risk categories.
Aid in Research
Co-author Dr. Christian Martin-Gill, the Chief of the Emergency Medical Services (EMS) division at UPMC, explains that the algorithm will significantly enhance the ability of EMS personnel and emergency department providers to identify individuals experiencing a heart attack or reduced blood flow to the heart. This improvement surpasses the capabilities of traditional ECG analysis.
Dr. Martin-Gill emphasized that this valuable information can guide crucial medical decisions made by EMS teams, such as initiating specific treatments in the field or notifying hospitals about the imminent arrival of a high-risk patient. Furthermore, he expressed excitement about the algorithm’s ability to identify low-risk patients who may not require transportation to a specialized cardiac facility, thereby potentially improving the prehospital triage process.
Dr. Al-Zaiti mentioned their efforts as a part of their upcoming developments in the research to formulate a cloud-based system that integrates with hospital command centers, enabling real-time analysis of ECG readings received from Emergency Medical Services (EMS). The model will then provide a risk assessment of the patient, thereby assisting medical professionals in making more precise, timely and informed decisions.