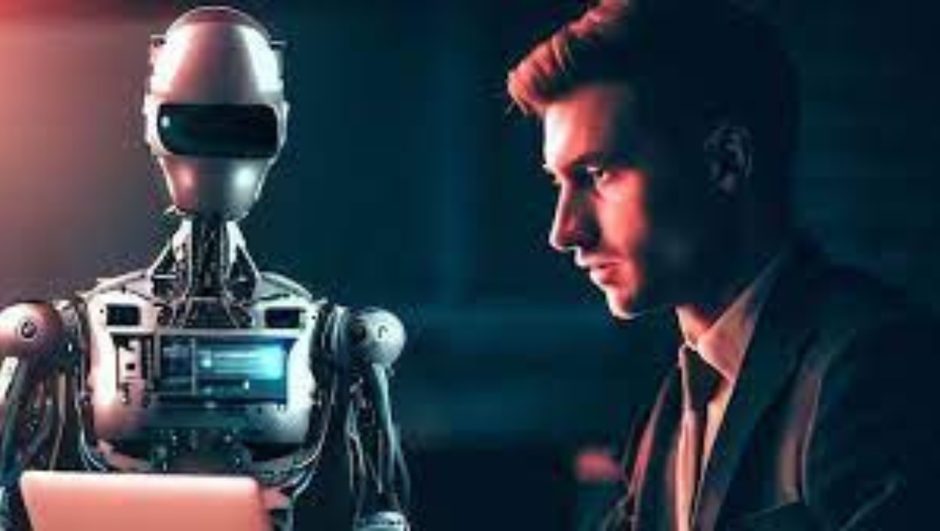
A deep literacy model can steal sensitive information like usernames, watchwords and dispatches by harkening to what you class on your keyboard. Trained by a platoon of experimenters from British universities, the sound – recognising algorithm can capture and decrypt keystrokes recorded from a microphone with 95 percent delicacy. According to Bleeping Computer, when the model was tested with the popular videotape conferencing results Zoom and Skype, the delicacy dropped to 93 per cent and 91.7 per cent.
The algorithm sheds light on how deep literacy can be potentially used to develop new types of malware which can hear to keyboard strokes to steal information like credit card figures, dispatches, exchanges and other particular information. The recent advancements in machine literacy combined with the vacuity of cheap high – quality microphones in the request make sound- grounded attacks more feasible compared to other styles that are frequently limited by factors like data transfer speed and distance.
To train the sound- recognising algorithm, the experimenters captured data by pressing 3 6 keys on a MacBook Pro 2 5 times each and recording the sound produced by those keys. The audio was captured using an iPhone 1 3 mini that was 1 7 cm down from the laptop.
The distinguished keys were produced from the waveforms and spectrograms. The distinct sound of each button was also used to train an image classifier called ‘ CoAtNet ’, which prognosticated which key was pressed on the keyboard. Still, the fashion doesn’t inescapably bear access to the device microphone. trouble actors can also join a drone call as a party to hear to keystrokes from druggies and infer what they’re codifying. According to the exploration paper, druggies can cover themselves from similar attacks by changing their typing patterns or using complex arbitrary watchwords.
How does deep learning work?
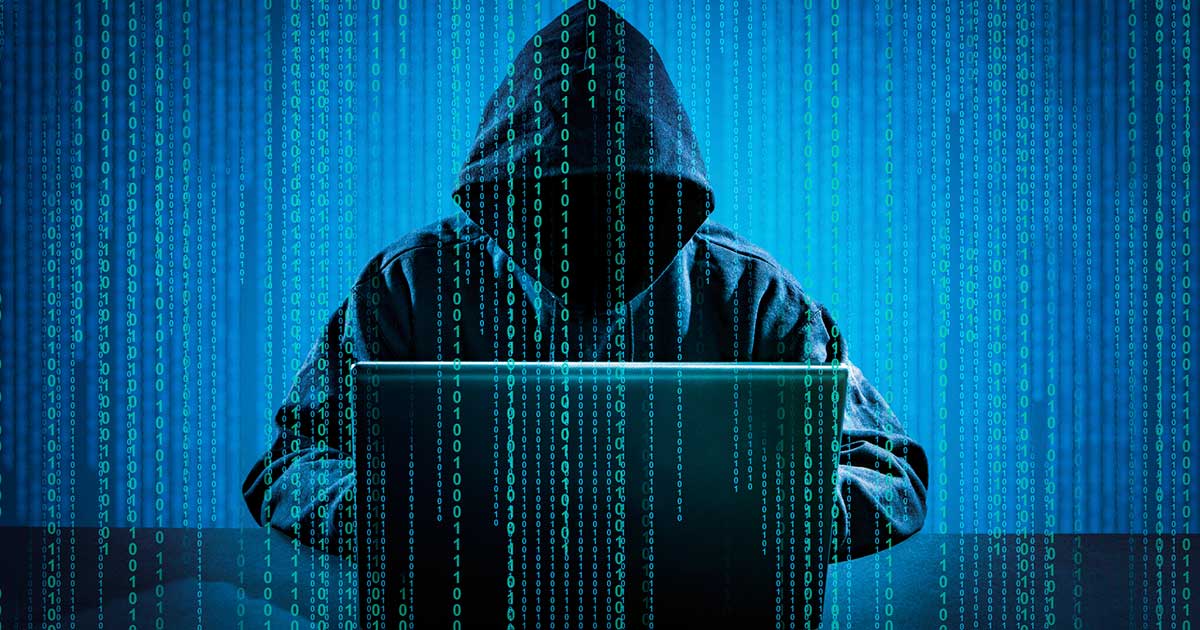
The white noise can also be used to make the model less accurate. Since the model was largely accurate on keyboards used by Apple on laptops in the last two times, which are generally silent, it’s largely doubtful that switching to silent would help. At present , the stylish way to deal with similar sound- grounded attacks is using biometric authentication like a point scanner, face recognition or an iris scanner.
Tapping in a computer word while drooling over drone could open the door to acyber – attack, exploration suggests, after a study revealed artificial intelligence( AI ) can work out which keys are being pressed by wiretapping on the sound of the typing. Experts say that as videotape conferencing tools similar as Zoom have grown in use, and bias with erected- in microphones have come ubiquitous, the trouble of cyber – attacks grounded on sounds has also risen. Now experimenters say they’ve created a system that can work out which keys are being pressed on a laptop keyboard with further than 9 0 delicacy, just grounded on sound recordings.
With smart bias bearing microphones getting ever more common within homes, similar attacks punctuate the need for public debates on governance of A I. The exploration, published as part of the IEEE European Symposium on Security and sequestration Workshops, reveals how Toreini and associates used machine literacy algorithms to produce a system suitable to identify which keys were being pressed on a laptop grounded on sound – an approach that experimenters stationed on the Enigma cipher device in recent times.
The study reports how the experimenters pressed each of 3 6 keys on a MacBook Pro, including all of the letters and figures, 2 5 times in a row, using different fritters and with varying pressure. The sounds were recorded both over a drone call and on a smartphone placed a short distance from the keyboard. The platoon also fed part of the data into a machine literacy system which, over time, learned to honor features of the aural signals associated with each key.
The system was also tested on the rest of the data. The results reveal that the system could directly assign the correct key to a sound 95 of the time when the recording was made over a phone call, and 93 of the time when the recording was made over a drone call.